概述
MetaGPT[1]是一个智能体开发框架,其功能完备,易于介入开发,而且是国内的团队开发的,直接可以对标微软的autogen等一众智能体框架。
MetaGPT集成了llama_index,进而实现了RAG,结合MetaGPT可以方便快速的接入自定义LLM,使得使用体验是很好的,比在llama_index中自定义LLM及使用RAG要更方便。
RAG使用
以官方的示例项目为例,跑一个rag_pipeline的程序。
安装
我个人是建议源码安装:pip install -e .[rag]
;可以方便快捷的改动源码调试;参考:RAG模块[2]。
向量模型部署
基于modelscope快速的安装Ollama,ollama官网的安装下载很慢,但可以基于ModelScope来下载安装,地址:Ollama-Linux[3]。下载后,拉取bge-m3向量模型,并指定环境变量运行:
# 拉取
OLLAMA_HOST=0.0.0.0:6006 ollama pull bge-me:567m
# 启动
CUDA_VISIBLE_DEVICES=2,3 OLLAMA_HOST=0.0.0.0:6006 ./ollama serve
如此,就将向量模型部署起来了。
MetaGPT本地LLM&RAG配置
一直没有在官方和网上找到如何配置本地的LLM&RAG,基本都是默认的openai类型。自己研究了下,按如下配置即可:
# Full Example: https://github.com/geekan/MetaGPT/blob/main/config/config2.example.yaml
# Reflected Code: https://github.com/geekan/MetaGPT/blob/main/metagpt/config2.py
# Config Docs: https://docs.deepwisdom.ai/main/en/guide/get_started/configuration.html
llm:
api_type: "open_llm" # or azure / ollama / groq etc.
model: "glm4" # or gpt-3.5-turbo
base_url: "http://127.0.0.1:7860/v1" # or forward url / other llm url
# max_token: 6000
# api_key: "empty"
# RAG Embedding.
# For backward compatibility, if the embedding is not set and the llm's api_type is either openai or azure, the llm's config will be used.
embedding:
api_type: "ollama" # openai / azure / gemini / ollama etc. Check EmbeddingType for more options.
base_url: "http://127.0.0.1:6006"
# api_key: ""
model: "bge-m3:567m"
dimensions: "1024" # output dimension of embedding model
RAG示例项目
接下来,运行官方的RAG示例项目:rag_pipeline.py,且注释掉es的代码:
async def main():
"""RAG pipeline.
Note:
1. If `use_llm_ranker` is True, then it will use LLM Reranker to get better result, but it is not always guaranteed that the output will be parseable for reranking,
prefer `gpt-4-turbo`, otherwise might encounter `IndexError: list index out of range` or `ValueError: invalid literal for int() with base 10`.
"""
# 解决 ValueError: Calculated available context size -12792 was not non-negative. 报错的问题
Settings._prompt_helper = PromptHelper(context_window=6000)
e = RAGExample(use_llm_ranker=False)
await e.run_pipeline()
await e.add_docs()
await e.add_objects()
await e.init_objects()
await e.init_and_query_chromadb()
# 暂时注释ES
# await e.init_and_query_es()
官方文档也提过可能会有ValueError: Calculated available context size -12792 was not non-negative
的报错,我也遇到了,本质上是集成的llama_index抛出来的,有两种方式一个是官方推荐的max_token
,我用的另一个:Settings._prompt_helper = PromptHelper(context_window=6000)
。
最终的运行日志如下:
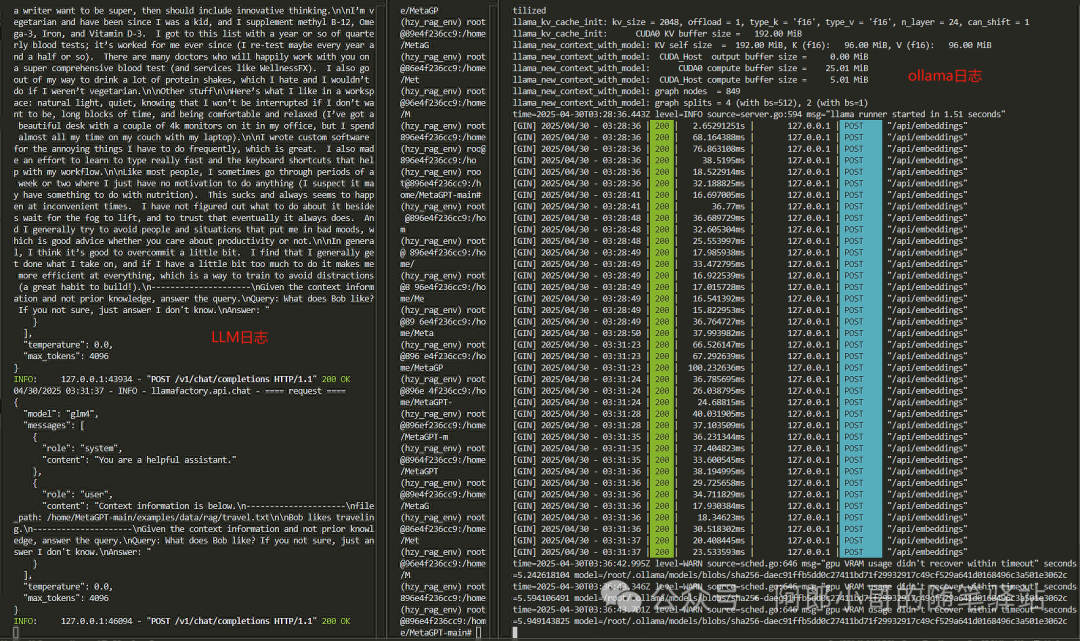
小结
其实整个流程没什么难点,主要是在配置项的确是没有参考的,也不知道是真没有基于本地LLM&RAG来配置的,还是采用的离线调用;我反正是比较能喜欢远程API的方式。
MetaGPT的RAG模块功能,是基于llama_index来实现的,其实就是直接集成进来的。因此对于RAG有需要优化的地方,是可以直接改源码的,这也是我推荐基于源码安装的原因。不过跟了一下llama_index的源码,我觉得还是写得蛮好的,尤其是现在基于event编排RAG流程。