在AI技术飞速发展的今天,图片RAG(Retrieval-Augmented Generation,检索增强生成)正逐渐成为多模态应用的“杀手锏”。无论是电商平台的“以图搜商品”,还是教育领域的“文本生成插图”,图片RAG通过检索与生成的高效结合,能带来令人惊叹的效果。
你是否遇到这样一个场景:
-
你在电商平台上传一张衣服的图片,系统不仅能找到相似款,还能自动生成搭配建议;
-
你在学习一篇复杂的医学文章,AI能智能匹配相关医学图像,甚至自动生成图文结合的讲解;
-
设计师上传一张草图,AI能检索相关风格图像,并生成更符合设计思路的创意草图。
这些神奇的功能背后,正是图片RAG的强大之处。它通过 检索+生成 的方式,既能保证结果的精准性,又能提升内容的创造力,让AI的理解能力跃升至全新高度。本篇文章都将为你提供 详细的实操指南、完整的代码示例,帮助你快速上手,打造一个 高性能的图片RAG系统!
一、什么是图片RAG?
简单来说,图片RAG是一种将图像检索与生成模型结合的技术。它的核心思想是:先从海量数据中检索出与用户输入最相关的图像或信息,再将这些检索结果作为上下文,输入到生成模型中,输出高质量的响应。有的场景直接从海量数据中检索出与用户输入最相关的图像或信息,图片RAG相比传统的单一检索或生成技术,图片RAG的优势在于:
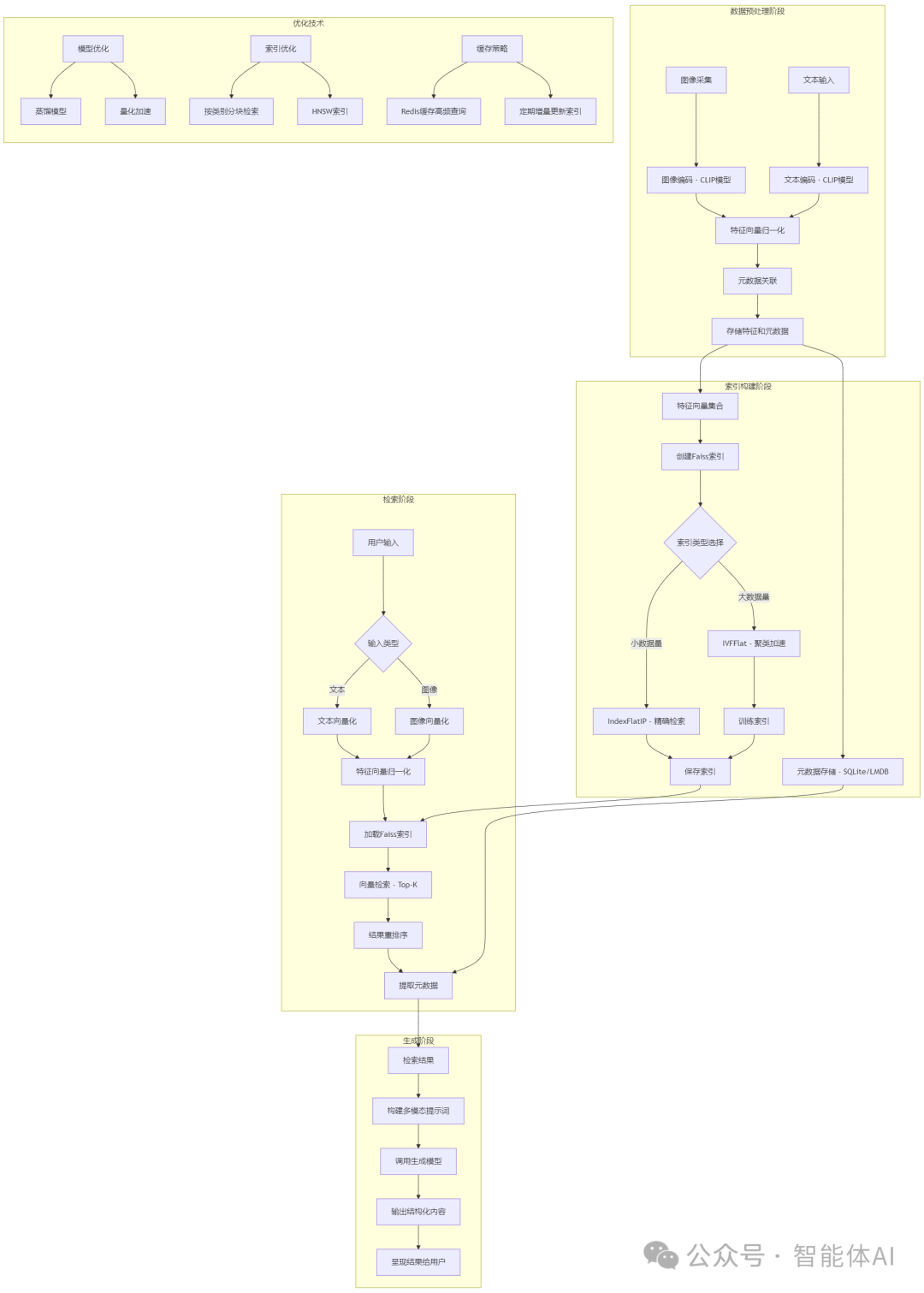
-
精准性:检索环节确保结果与用户输入高度相关。
-
创造性:生成模型能进一步丰富输出内容。
-
多模态:支持文本、图像等多种输入形式。
接下来,我们将按技术流程拆解图片RAG的每个环节,并提供详细的实现步骤和代码,确保你能直接上手实践。
二、数据预处理与特征提取:打好基础
1. 图像编码:从像素到向量
图片RAG的第一步是将图像转化为机器能理解的特征向量。我们推荐使用CLIP模型(ViT-B/32),这是由OpenAI开发的一个强大工具,能够将图像和文本映射到同一个向量空间,非常适合多模态任务。
工具选择:
-
模型:CLIP(ViT-B/32),因其出色的图像-文本对齐能力。
-
框架:Hugging Face的transformers库,简单易用且社区支持广泛。
前置准备:
确保你已安装必要的库:
pip install torch transformers pillow numpy
代码实现:
以下是一个完整的图像特征提取示例,确保你有一张名为image.jpg的图片在工作目录下:
from PIL import Image
from transformers import CLIPProcessor, CLIPModel
import numpy as np
# 加载预训练的CLIP模型和处理器
model = CLIPModel.from_pretrained("openai/clip-vit-base-patch32")
processor = CLIPProcessor.from_pretrained("openai/clip-vit-base-patch32")
# 打开并处理图像
image = Image.open("image.jpg").convert("RGB") # 确保图像为RGB格式
inputs = processor(images=image, return_tensors="pt", padding=True) # 转为PyTorch张量
# 提取图像特征
image_features = model.get_image_features(**inputs).detach().numpy()
# 归一化特征向量
image_features = image_features / np.linalg.norm(image_features, axis=1, keepdims=True)
# 检查结果
print("图像特征维度:", image_features.shape) # 应为 (1, 512)
print("图像特征示例:", image_features[0][:5]) # 查看前5个值
注意事项:
-
归一化重要性:归一化将特征向量的长度统一为1,避免因向量大小差异影响后续检索的余弦相似度计算。
-
异常处理:如果图像打不开或格式错误,建议添加try-except块,确保代码健壮性:
try: image = Image.open("image.jpg").convert("RGB")except Exception as e: print(f"图像加载失败: {e}") exit(1)
2. 文本对齐:图文合一
如果你的应用场景需要支持文本查询(如“红色连衣裙”),需要将文本转为向量,与图像特征对齐。CLIP的强大之处在于它能同时处理图像和文本。
代码实现:
# 定义文本查询
text = "a red dress"
text_inputs = processor(text=text, return_tensors="pt", padding=True)
# 提取文本特征
text_features = model.get_text_features(**text_inputs).detach().numpy()
# 归一化文本特征
text_features = text_features / np.linalg.norm(text_features, axis=1, keepdims=True)
# 检查结果
print("文本特征维度:", text_features.shape) # 应为 (1, 512)
print("文本特征示例:", text_features[0][:5]) # 查看前5个值
注意事项:
-
确保文本输入简洁,避免过长句子影响特征质量。
-
如果需要多语言支持,可尝试CLIP的多语言变体(如openai/clip-vit-base-patch32-multilingual)。
3. 元数据关联:业务与技术的桥梁
特征提取后,需要将特征向量与业务数据(如商品ID、价格)关联起来。我们推荐使用Pandas将数据保存为Parquet格式,既高效又节省磁盘空间。
前置准备:
安装Pandas:
pip install pandas pyarrow
代码实现:
import pandas as pd
# 构造元数据
metadata = {
"image_id": ["img_001"],
"feature": [image_features.tobytes()], # 转为二进制存储
"category": ["dress"],
"price": [299]
}
# 创建DataFrame并保存为Parquet
df = pd.DataFrame(metadata)
df.to_parquet("image_metadata.parquet", engine="pyarrow")
# 验证保存结果
print("元数据已保存至 image_metadata.parquet")
df_loaded = pd.read_parquet("image_metadata.parquet")
print(df_loaded)
注意事项:
-
二进制存储:tobytes()将NumPy数组转为二进制,节省空间且便于数据库存储。
-
批量处理:如果有大量图像,建议循环处理并追加到DataFrame中。
三、索引构建:让检索快如闪电
1. 向量索引:Faiss来帮忙
有了特征向量,下一步是构建索引以实现快速检索。我们推荐使用Faiss,这是Facebook开源的稠密向量检索库,支持GPU加速,效率极高。
前置准备:
安装Faiss(CPU版为例,GPU版需编译):
pip install faiss-cpu
假设你已提取了多张图像的特征,保存为all_image_features.npy(形状为(n, 512),n为图像数量):
# 示例:生成模拟特征import numpy as npnp.random.seed(42)all_image_features = np.random.randn(1000, 512).astype(np.float32) # 1000张图像np.save("all_image_features.npy", all_image_features)
基础索引:
import faiss
# 定义特征维度
dim = 512 # CLIP特征维度
# 创建内积相似度索引
index = faiss.IndexFlatIP(dim)
# 加载特征并添加至索引
features = np.load("all_image_features.npy")
index.add(features)
# 保存索引
faiss.write_index(index, "image_index.faiss")
# 验证索引
print("索引中的向量总数:", index.ntotal) # 应为1000
优化索引:IVFFlat提速
对于百万级数据,IndexFlatIP搜索速度较慢,推荐使用IVFFlat(倒排文件索引)通过聚类减少搜索范围。
# 定义聚类中心数
nlist = 100 # 根据数据量调整,建议为sqrt(n)
quantizer = faiss.IndexFlatIP(dim)
# 创建IVFFlat索引
index = faiss.IndexIVFFlat(quantizer, dim, nlist, faiss.METRIC_INNER_PRODUCT)
# 训练索引
index.train(features)
# 添加特征
index.add(features)
# 保存索引
faiss.write_index(index, "image_index_ivf.faiss")
# 设置搜索范围(可选)
index.nprobe = 10 # 搜索10个聚类中心,平衡速度与精度
注意事项:
-
nlist选择:数据量越大,nlist应越大,但过大会增加训练时间。
-
GPU加速:若有GPU,可安装faiss-gpu,只需将IndexFlatIP替换为GpuIndexFlatIP。
2. 元数据存储:SQLite上场
检索时,除了向量,还需返回业务信息。我们用SQLite存储图像ID、特征和元数据。
前置准备:
SQLite无需额外安装,Python自带支持。
代码实现:
import sqlite3
# 连接数据库
conn = sqlite3.connect("image_rag.db")
# 创建表
conn.execute('''CREATE TABLE IF NOT EXISTS images
(id TEXT PRIMARY KEY, feature BLOB, category TEXT, price INT)''')
# 插入示例数据
conn.execute("INSERT OR REPLACE INTO images VALUES (?, ?, ?, ?)",
("img_001", image_features.tobytes(), "dress", 299))
# 提交更改
conn.commit()
# 验证数据
cursor = conn.cursor()
cursor.execute("SELECT * FROM images WHERE id='img_001'")
print("查询结果:", cursor.fetchone())
conn.close()
注意事项:
-
主键唯一性:id设为TEXT PRIMARY KEY,避免重复插入。
-
批量插入:若数据量大,可用executemany提高效率。
四、检索阶段:找到最匹配的内容
1. 处理用户输入
用户可能输入文本(如“蓝色衬衫”)或图像,我们需要统一转为向量。
文本查询:
text = "a blue shirt"text_inputs = processor(text=text, return_tensors="pt", padding=True)text_features = model.get_text_features(**text_inputs).detach().numpy()text_features = text_features / np.linalg.norm(text_features, axis=1, keepdims=True)
图像查询:
query_image = Image.open("query.jpg").convert("RGB")query_inputs = processor(images=query_image, return_tensors="pt", padding=True)query_features = model.get_image_features(**query_inputs).detach().numpy()query_features = query_features / np.linalg.norm(query_features, axis=1, keepdims=True)
2. 执行检索
用Faiss检索Top-K结果,再从SQLite拉取元数据。
代码实现:
# 加载索引
index = faiss.read_index("image_index_ivf.faiss")
# 检索Top-5结果
k = 5
D, I = index.search(query_features, k) # D为距离,I为索引
# 连接数据库
conn = sqlite3.connect("image_rag.db")
cursor = conn.cursor()
# 获取元数据
results = []
for idx in I[0]:
cursor.execute("SELECT id, category, price FROM images WHERE rowid=?", (idx + 1,))
results.append(cursor.fetchone())
conn.close()
# 输出结果
print("检索结果:")
for result in results:
print(f"ID: {result[0]}, Category: {result[1]}, Price: {result[2]}")
注意事项:
-
rowid偏移:Faiss索引从0开始,而SQLite的rowid从1开始,故需idx + 1。
-
异常处理:若索引或数据库为空,需添加检查。
3. 重排序(可选)
若对精度要求更高,可用交叉编码器对结果精排。
前置准备:
pip install sentence-transformers
代码实现:
from sentence_transformers import CrossEncoder
# 加载交叉编码器
reranker = CrossEncoder("cross-encoder/ms-marco-MiniLM-L-6-v2")
# 假设检索结果有描述文本
result_descriptions = ["Red silk dress", "Blue cotton shirt"]
query_text = "a blue shirt"
pairs = [(query_text, desc) for desc in result_descriptions]
# 计算相关性得分
scores = reranker.predict(pairs)
# 按得分排序
sorted_indices = np.argsort(scores)[::-1]
print("重排序后索引:", sorted_indices)
五、生成阶段:从检索到创意输出
1. 多模态提示词
将用户查询和检索结果组合成提示词,交给生成模型。
代码实现:
user_query = "a blue shirt"prompt = f"""User Query: {user_query}Retrieved Images: [img_001.jpg, img_002.jpg] (Categories: dress, shirt)Retrieved Text: "This blue shirt is made of cotton, priced at $49."Task: Generate a response explaining why these results are relevant."""
2. 调用生成模型
由于gpt-4非开源,我们用EleutherAI/gpt-neo-1.3B作为示例。
前置准备:
pip install transformers
代码实现:
from transformers import pipeline
# 加载生成模型
generator = pipeline("text-generation", model="EleutherAI/gpt-neo-1.3B")
# 生成响应
response = generator(prompt, max_length=200, num_return_sequences=1)
print("生成结果:", response[0]["generated_text"])
注意事项:
-
模型选择:若需更强效果,可用付费API(如OpenAI GPT-4)。
-
长度控制:max_length根据需求调整。
3. 输出结构化
想生成JSON格式?在提示词中指定即可。
代码实现:
prompt += "nFormat the response as JSON with keys: 'product_id', 'reason'."response = generator(prompt, max_length=200)print("结构化输出:", response[0]["generated_text"])
六、效率优化:让系统更快更强
1. 模型优化
蒸馏模型:
使用轻量版CLIP:
model = CLIPModel.from_pretrained("asus-uwk/distil-clip-vit-base-patch32")
量化加速:
from torch.quantization import quantize_dynamicmodel = quantize_dynamic(model, {torch.nn.Linear}, dtype=torch.qint8)
2. 索引优化
按类别分块检索:
cursor.execute("SELECT category FROM images WHERE id=?", ("img_001",))category = cursor.fetchone()[0]sub_index = faiss.read_index(f"indices/{category}.faiss")D, I = sub_index.search(query_features, k=5)
3. 缓存策略
使用Redis缓存高频查询:
前置准备:
pip install redis# 启动Redis服务器(需本地安装Redis)redis-server
代码实现:
import redis
import json
r = redis.Redis(host="localhost", port=6379, db=0)
cache_key = f"retrieval:{hash(str(query_features))}"
if r.exists(cache_key):
results = json.loads(r.get(cache_key))
else:
D, I = index.search(query_features, k=5)
results = [{"id": i} for i in I[0]] # 简化示例
r.setex(cache_key, 86400, json.dumps(results)) # 缓存24小时
print("缓存结果:", results)
七、端到端案例:电商以图搜商品
1. 数据准备
-
图像路径:/tanjp/data/products/*.jpg
-
元数据:product_id, image_path, category, price, description
2. 批量特征提取
保存为extract_features.py:
import argparse
import glob
from PIL import Image
import numpy as np
parser = argparse.ArgumentParser()
parser.add_argument("--input_dir", default="/tanjp/data/products")
parser.add_argument("--output", default="features.npy")
args = parser.parse_args()
images = glob.glob(f"{args.input_dir}/*.jpg")
features = []
for img_path in images:
image = Image.open(img_path).convert("RGB")
inputs = processor(images=image, return_tensors="pt")
feat = model.get_image_features(**inputs).detach().numpy()
features.append(feat[0])
np.save(args.output, np.array(features))
运行:
python extract_features.py --input_dir /tanjp/data/products --output features.npy
3. 服务部署(FastAPI)
前置准备:
pip install fastapi uvicorn
代码实现:
from fastapi import FastAPI, File, UploadFile
import io
app = FastAPI()
async def search(image: UploadFile = File(...)):
img_bytes = await image.read()
query_image = Image.open(io.BytesIO(img_bytes)).convert("RGB")
inputs = processor(images=query_image, return_tensors="pt")
query_features = model.get_image_features(**inputs).detach().numpy()
query_features = query_features / np.linalg.norm(query_features, axis=1, keepdims=True)
D, I = index.search(query_features, k=5)
return {"results": [int(i) for i in I[0]]}
运行:
uvicorn main:app --host 0.0.0.0 --port 8000
4. 前端调用
<input type="file" id="imageInput" accept="image/*">
<button onclick="search()">Search</button>
<div id="results"></div>
<script>
async function search() {
const file = document.getElementById("imageInput").files[0];
const formData = new FormData();
formData.append("image", file);
const response = await fetch("http://localhost:8000/search", {
method: "POST",
body: formData
});
const data = await response.json();
document.getElementById("results").innerText = JSON.stringify(data.results);
}
</script>
八、常见问题解决
在构建图片RAG系统时,我们可能会遇到一些 性能瓶颈、数据管理、索引优化 等问题。以下是几个常见问题及解决方案。
1. 内存占用过高,如何优化?
问题分析:
-
存储大量高维特征向量时,内存需求可能会飙升。
-
检索时如果载入所有数据,容易导致 OOM(Out of Memory)。
解决方案:
-
使用 LMDB 进行高效存储:LMDB(Lightning Memory-Mapped Database)是一个高性能的键值存储数据库,适合存储大规模特征向量。
import lmdb
import numpy as np
# 创建LMDB数据库
env = lmdb.open("features.lmdb", map_size=10**12) # 1TB空间
# 存储特征向量
with env.begin(write=True) as txn:
txn.put("img_001".encode(), np.random.rand(512).astype(np.float32).tobytes())
-
分批加载索引:避免一次性加载所有数据,改为 按需加载。
2. 检索速度太慢,如何加速?
问题分析:
-
直接使用 IndexFlatIP 进行向量检索时,搜索时间会随数据量增长而变慢。
解决方案:
-
使用 IVFFlat 进行索引优化:
import faiss
# 512维特征,100个聚类中心
quantizer = faiss.IndexFlatIP(512)
index = faiss.IndexIVFFlat(quantizer, 512, 100, faiss.METRIC_INNER_PRODUCT)
index.train(np.random.rand(10000, 512).astype(np.float32))
index.add(np.random.rand(10000, 512).astype(np.float32))
-
使用 GPU 加速:如果数据量特别大,可以用 Faiss-GPU 版本 提高检索效率。
res = faiss.StandardGpuResources()index = faiss.GpuIndexFlatIP(res, 512)
3. 如何保证检索结果的业务可解释性?
问题分析:
-
纯粹基于向量匹配的检索,可能会出现 结果相关但不合理 的情况。
解决方案:
-
结合元数据进行后处理:检索后,对结果进行二次筛选,例如:
-
按 类别 过滤:衣服搜索结果里不应该混入鞋子。
-
按 价格 过滤:不显示超出预算的商品。
results = [r for r in retrieved_results if r["category"] == "clothing"]
九、应用场景:从创意到实用
1. 电商:以图搜商品 + 生成个性化推荐
-
用户上传一张衣服的照片,AI能:
-
检索 出相似款,并提供购买链接。
-
生成 商品推荐理由,如“这件衣服与您的风格相符,并有 20% 的折扣”。
2. 教育:智能插图生成
-
输入一段生物学教材,AI能:
-
检索 相关解剖图。
-
生成 一张符合教学需求的插图。
3. 设计创意:AI辅助艺术创作
-
?设计师上传一张草图,AI能:
-
检索 相似风格的参考图。
-
生成 进阶版本的设计方案。
十、效率提升的锦囊妙计
1. 向量检索优化
-
使用 HNSW 索引:比 IVFFlat 更适合 高维向量。
-
分层索引:先用 IVFFlat 粗筛,再用 IndexFlatIP 精筛,加速计算。
2. 生成模型加速
-
使用 DistilGPT-2 代替 GPT-4,减少计算开销。
-
量化模型:使用 torch.quantization 让模型计算更高效。
from torch.quantization import quantize_dynamicimport torch.nn as nnmodel = quantize_dynamic(model, {nn.Linear}, dtype=torch.qint8)
3. 业务落地优化
-
使用 Redis 缓存高频检索结果,减少重复计算。
-
定期增量更新索引,保持数据最新。
十一、总结
通过本文,你已经掌握了 图片RAG的完整技术路线,从 数据预处理 到 检索优化,再到 生成增强,我们提供了详细的实操指南,让你可以快速落地自己的 AI 应用。
无论你是想优化 电商搜索,提升 教育体验,还是赋能 创意设计,图片RAG都能帮你大幅提升 AI 生成的智能性和实用性。